Physics-Informed Neural Networks for Structural Dynamics
ABG-132123 | Sujet de Thèse | |
21/05/2025 | Contrat doctoral |
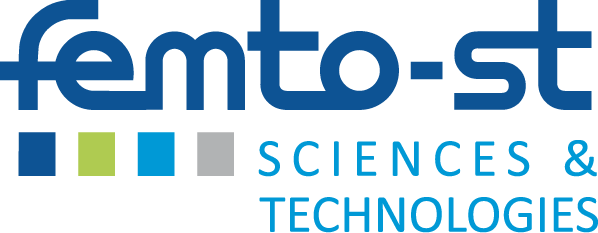
- Sciences de l’ingénieur
Description du sujet
Physics-Informed Neural Networks (PINNs) have emerged as a paradigm for solving forward and inverse problems in computational mechanics by integrating physical laws directly into the learning process. Recently reintroduced by Raissi et al. (2019), PINNs enforce governing equations, typically partial differential equations (PDEs), as constraints in the neural network loss function, enabling the use of sparse or indirect data to infer system behavior.
In structural dynamics and vibroacoustics, which deal with the response of mechanical systems to dynamic loading, the accurate prediction and identification of structural behavior are often computationally intensive. These problems involve solving complex-valued formulations of elastodynamic and acoustic PDEs. Conventional solvers like the finite element method (FEM) face limitations due to meshing costs, high-dimensional parameter spaces, and sensitivity to input uncertainty, especially in inverse problems such as material identification, force reconstruction, or damage localization.
Recent studies have demonstrated the applicability of PINNs to dynamic problems in solid and structural mechanics. Chen and Ghaboussi (2022) proposed a PINN-based approach for inverse problems in structural dynamics, showing that PINNs can infer boundary conditions and system parameters from vibrational responses. Haghighat et al. (2022) tackled frequency-domain wave propagation using PINNs and identified critical challenges such as accuracy degradation at high frequencies. Extensions of the framework, such as complex-valued PINNs or frequency-domain formulations (Zhang et al., 2023), have shown promise in addressing these challenges by adapting the architecture and loss formulation to better capture oscillatory solutions. More recently, a significant step forward has been taken with the possibility of identifying non-homogeneous properties and even damping properties of heterogeneous beams (Teloli et al., 2025).
In the context of inverse problems, PINNs have shown strong performance in recovering unknown fields (e.g., material parameters or input forces) using only partial observations. Rao et al. (2021), Xu et al. (2021), Teloli et al. (2025) developed PINN models for dynamic elastodynamic inverse problems, material characterization, and damping identification, respectively. These works highlight the capacity of PINNs to generalize across different loading conditions and frequencies, making them particularly suited for structural health monitoring and system identification in complex systems.
The proposed PhD research aims to extend the capabilities of PINNs in structural dynamics and vibroacoustic problems. This includes:
• Developing complex-valued PINN architectures tailored for frequency-domain PDEs,
• Addressing high-frequency limitations through domain decomposition, adaptive sampling, or hybrid methods combining PINNs with traditional solvers,
• Applying these methods to compute vibroacoustic responses and/or identify unknown inputs (e.g., forces, boundary conditions) and system parameters (e.g., stiffness, damping) using sparse frequency response data.
The outcomes of this research are expected to contribute to more efficient and interpretable modeling tools for structural dynamics and acoustic systems, with applications ranging from aerospace and automotive engineering to built environment diagnostics.
References
• Raissi, M., Perdikaris, P., & Karniadakis, G. E. (2019). Physics-informed neural networks: A deep learning framework for solving forward and inverse problems involving nonlinear partial differential equations. Journal of Computational Physics, 378, 686–707. https://doi.org/10.1016/j.jcp.2018.10.045
• Rao, C., Sun, L., & Darve, E. (2021). Physics-informed deep learning for computational elastodynamics without labeled data. Journal of Computational Physics, 443, 110525. https://doi.org/10.1016/j.jcp.2021.110525
• Xu, K., Wang, S., & Perdikaris, P. (2021). Learning constitutive relations of nonlinear solid mechanics from data with physics-informed neural networks. Computer Methods in Applied Mechanics and Engineering, 383, 113896. https://doi.org/10.1016/j.cma.2021.113896
• Haghighat, E., Juanes, R., & Gomez, H. (2022). PINNs for wave propagation: Can physics-informed deep-learning replace traditional solvers? Journal of the Mechanics and Physics of Solids, 164, 104871. https://doi.org/10.1016/j.jmps.2022.104871
• Chen, Y., & Ghaboussi, J. (2022). Physics-informed neural networks for inverse problems in structural dynamics. Engineering Structures, 253, 113720. https://doi.org/10.1016/j.engstruct.2021.113720
• Zhang, R., Sun, H., & Karniadakis, G. E. (2023). Complex-valued physics-informed neural networks for frequency-domain wave propagation problems. Computer Methods in Applied Mechanics and Engineering, 411, 115503. https://doi.org/10.1016/j.cma.2023.115503
• Teloli, R. D. O., Tittarelli, R., Bigot, M., Coelho, L., Ramasso, E., Le Moal, P., & Ouisse, M. (2025). A physics-informed neural networks framework for model parameter identification of beam-like structures. Mechanical Systems and Signal Processing, 224, 112189. https://doi.org/10.1016/j.ymssp.2024.112189
Prise de fonction :
Nature du financement
Précisions sur le financement
Présentation établissement et labo d'accueil
See website
Site web :
Intitulé du doctorat
Pays d'obtention du doctorat
Etablissement délivrant le doctorat
Ecole doctorale
Profil du candidat
Candidates must have received the grade of MSc or Engineer in Mechanics, and have a background in Machine Learning algorithms.
Vous avez déjà un compte ?
Nouvel utilisateur ?
Vous souhaitez recevoir nos infolettres ?
Découvrez nos adhérents
Nokia Bell Labs France
SUEZ
MabDesign
CESI
PhDOOC
ASNR - Autorité de sûreté nucléaire et de radioprotection - Siège
Institut Sup'biotech de Paris
TotalEnergies
ADEME
Généthon
ANRT
CASDEN
Ifremer
MabDesign
Laboratoire National de Métrologie et d'Essais - LNE
Aérocentre, Pôle d'excellence régional
Tecknowmetrix
ONERA - The French Aerospace Lab
Groupe AFNOR - Association française de normalisation